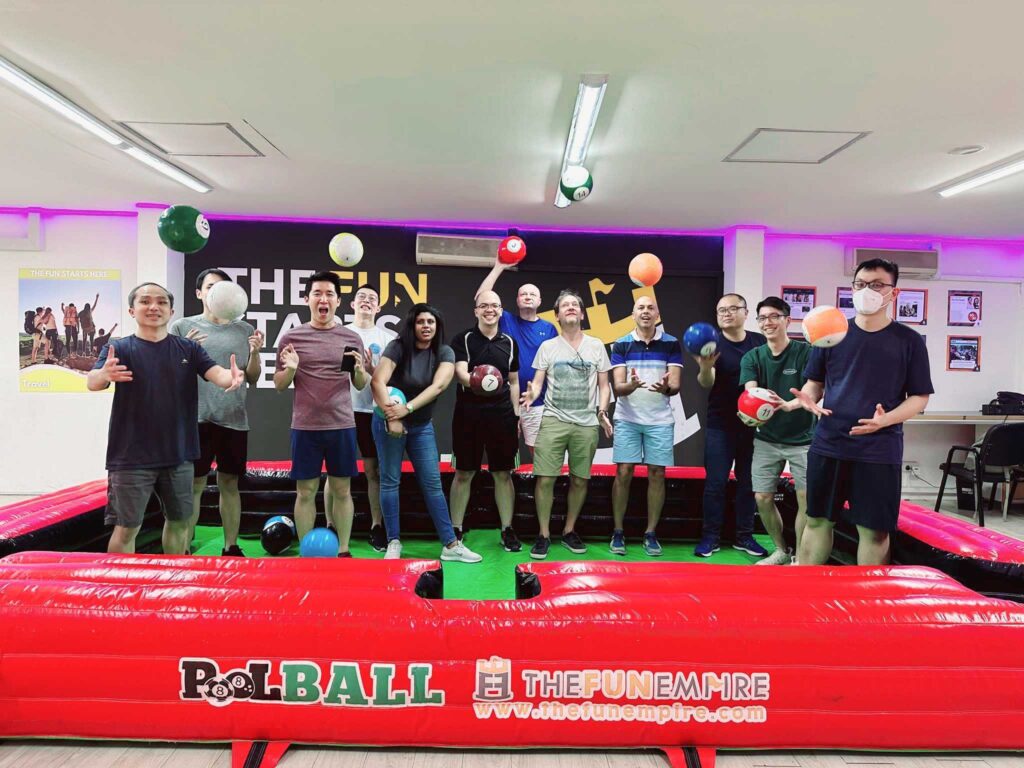
Founders: Xavier Conort & Razi Raziuddin
Founding: 2022
Mission: Scale enterprise AI, by radically simplifying and industrializing AI data
Employees: 15 & 25% Local
Workplace: Remote
Stage & Capital Raised: Seed & $5.7M raised
Investors: Glasswing Ventures & Tola Capital
Key Customers: TBD
Glassdoor Rating: N/A
Valuation (estimated): <$50M
^ this is a useless number. There is no tangible valuation until the business is sold or goes public. Don’t forget it!
FeatureByte is a “modern AI data platform for data scientists”. They are on a mission to scale enterprise AI by building a SaaS solution that helps simplify the creation, serving, management and monitoring of machine learning features. Founded in 2022 by Xavier Conort & Razi Raziuddin, the FeatureByte team knows data science and machine learning.
Razi, Xavier & much of the early FeatureByte team met at Boston based unicorn DataRobot. Razi was DataRobot’s first business hire and helped scale the company from 10 to 1,000 employees in under 6 years. He departed as their SVP of AI Services. Previously, Razi worked at Netezza before it was acquired by IBM, managing Product Marketing. Xavier was most recently DataRobot’s Chief Data Scientist. FeatureByte Customer 0, perhaps? Perhaps we should reiterate, this team knows data science and machine learning.
They understand intimately that BI is for humans and the AI wave will be for machines. Razi adds “the best way to think about AI versus BI is in terms of the ultimate consumer of data. BI metrics are primarily designed for human consumption and are descriptive in nature. Whereas AI features are intended for consumption by machines and algorithms. They are at least an order of magnitude larger in number and more computationally complex. Hence the complexity of managing the data pipelines and infrastructure associated with AI versus BI”.
In the last decade, a lot has changed in the way that machine learning tools (ahem, AI) are developed. The modern data and analytics stack is built to mostly serve BI workflows. AI data management is different. For one, it’s more complex. You need faster tools that can handle rapid experimentation, complex computations, historical accuracy, and help with segmentation to prevent machine learning’s time travel problem (blocking future signals from informing historical training data). The global AutoML and MLOps market is expected to grow to more than $25 billion by 2028.
The FeatureByte team is building a Feature Engineering & Management platform to empower data scientists to create and share state-of-the-art features and production-ready data pipelines in minutes – instead of weeks or months. Their solution is meant to handle the entire end to end lifecycle of machine learning feature development that is happening across every industry, including help with managing cost & complexity after deployment. Heading into 2024, their focus will be on automating product capabilities by incorporating Generative AI capabilities. From a business perspective, it’ll be all about expanding the user base and use cases they can help with.
Most recently, FeatureByte released their open source SDK in May so that any data scientist can get started for free. Using Python, any user can create state of the art features and deploy feature pipelines in minutes with just a few lines of code. The SDK automatically generates complex, time-aware SQL to perform feature transformations at scale in cloud data platforms such as Databricks and Snowflake. This self-service data environment for data scientists allows organizations to realize iterations on live data, cleaner data to make better business decisions, and productivity gains to deliver compute efficiency.
Operators to Know (Locally):
- Hanna McLean, Marketing Manager
- Stephen Millet, Principal Software Engineer
- Razi Raziuddin, Co-Founder & CEO
- Sergey Yurgenson, Head of Semantec Data Science
My investigative powers continue to need work so apologies to the FeatureByte team I’m sure I missed some up & coming operators internally
Key Roles To Be Hired:
- More roles coming soon in the months ahead!
If I were interviewing here are some questions I’d ask:
- What are the key milestones FeatureByte is looking to achieve in 2023?
- How does the explosion in LLM (large language model) development change the GTM approach for the company?
- What are the biggest challenges as you scale out the initial team and functions?
- What is the long term vision for the company?
We’re optimizing for readability here so to learn more about FeatureByte you’ll have to D.Y.O.R. I’m excited to watch this team bring more data scientists into the machine learning age. All technical storytellers applaud your efforts. See you around town!